Skyral People Team
Behind the Screen: Meet Lead Applied Scientist Dr. Giuseppe Castegnetti
Skyral People Team
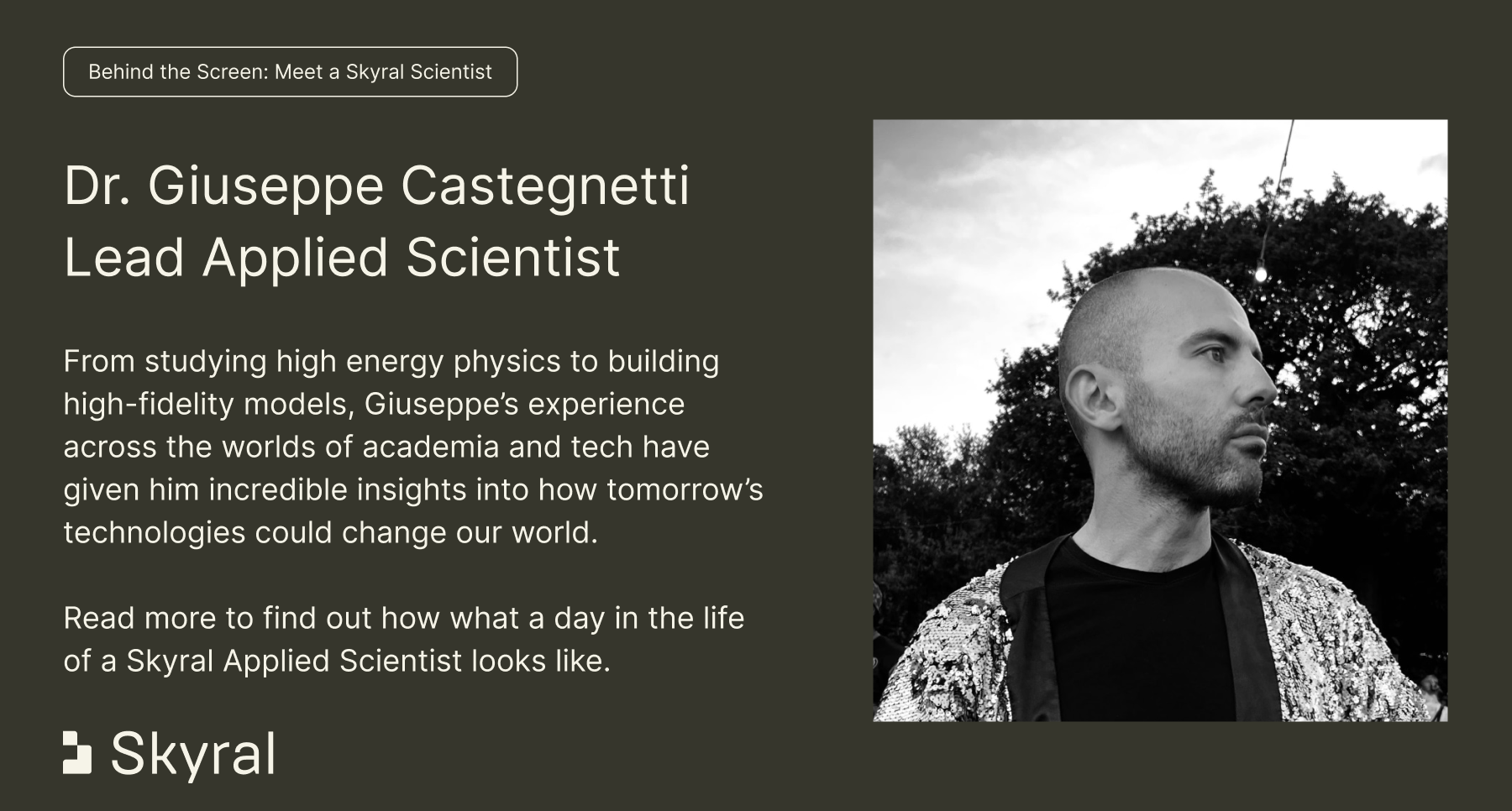
Career Journey & Job Role
Q: Tell me a bit about your career to date?
So Skyral (or when we were part of Improbable Defence) was my first job outside academia. I studied in Milan for my undergrad and my Masters, which I earned in physics. Then I moved to Zurich for my PhD in Neuroscience.
I made the switch from physics to neuroscience because my focus, which was high energy particle physics, was pushing me toward large scientific collaborations, because to study high energy physics, you need very expensive, very complicated machines to conduct experiments such that only large and often multinational collaborations can afford them. This means that the direction of work is set at a much higher level and I thought there was less room for a junior researcher to make an impact with new ideas.
Plus, as an over-confident young man, I was afraid to get lost in the sea of scientists that were working on the same project. Neuroscience was something that I found very interesting and at the same time could give me the opportunity to work more independently.
While in Zurich, I was able to define end-to-end experiments and produce results myself. My research brought me to London, for my post doc, and then Improbable Defence. Improbable Defence, now Skyral, caught my eye because of my interest in behavioural modelling. At the time, I was already working on decision-making and human behaviour modelling myself, as part of my research. So, that’s how I pivoted into tech.
On Life @ Skyral
Q: Take me through your average day as one of Skyral’s Lead Applied Scientists?
I am still mostly focussed on modelling human behaviour for social simulations, but now my work spans a number of projects and teams, so it tends to be more high-level compared to when I started. A big part of my day-to-day involves understanding the feasibility of how different models feed into, and support, our modelling projects to make sure we have the right combination of technologies to deliver for our customers.
Q: What’s the best part about working at Skyral?
We are trying to solve problems that people really aren’t solving right now. Call them: the ‘messy’ problems. They’re the ones that don’t fit into the categories of problems that you normally find in the research or tech industry these days. And that’s really exciting because we’re trying to combine a number of technologies, from AI to behavioural modelling, to distributed systems, and many more, to come up with something that is extremely complicated to create and understand, even in the age of AI. We don’t have an AI formula for most of the problems we are trying to solve. It’s a completely unique space to work in that is overdue and in high demand.
Q: What’s the biggest challenge of working at Skyral?
When it comes to my team’s work, we’re trying to gauge what will be most useful for our customers. While we try our best to understand our customers’ problems and challenges, our biggest challenge from a scientific and delivery point of view is building a technological landscape of tools that help them solve the problems that they perhaps aren’t even aware of yet, but that will be crucial to helping them improve their decision making and understand the implications their decisions into the future.
If you look at it directly from the modelling perspective, the challenge becomes, ‘how do I create a model that tells you something useful about your system today, will deliver deeper insights tomorrow, all while keeping the complexity of a model to a minimum?’
So take our military planner customers, for example. They have developed a great intuition of knowing what the consequences of their actions will be in a given crisis situation. Now, what we need to do with our technologies and models is to test scenarios in order to tell them the result of something that they don’tknow. For example, if there is an attack in central London, we know people are going to try and escape from London. Our planners have some intuition for what that will look like. However, there are gaps between that intuition and the complexity of the actual outcome. So we have to find those gaps between what they understand through experience and their own intuition, and what the capability of our models can predict – which go into 2nd, 3rd, and 4th order effects – well beyond what human intuition can gauge on its own.
It’s about getting the best insights and making better decisions through the right application of human-machine pairing. It’s both challenging and very exciting!
Q: What makes Skyral unique? How would you describe ‘Life @Skyral’?
I mean, the people are great. We have a great record of hiring good people, both from a professional perspective, sure, but also from a human perspective. Most people at some point in their working life have felt like they’ve been treated unfairly, or something unjust happens to them at work, but honestly I’ve never had an experience like that here.
I think that’s because the working environment, both contextually and culturally in the UK, and in tech, is much better than in other parts of the world. And at Skyal specifically, we’ve done such a good job of creating a ‘no blame’ culture. When you join a team of people who already have that type of culture ingrained, you really have to get on board with it. Kindness breeds kindness. If you’re not kind, you simply won’t fit.
On How to Train AI Models
Q: So, starting at the beginning, what is this modelling technology?
Well, first off, what we do is quite different from what people would normally call AI these days. While conventional AI systems primarily learn to replicate patterns from data, we develop models mostly based on theory and domain-specific principles, incorporating data-driven techniques as a complement, for example for model validation.
When we started doing this a few years ago, we were building on a long tradition of scientific research in our area of interest: behavioural economics, neuroscience, psychology, experimental psychology, the social sciences, etc. Scientists had already tried to understand in the past how individuals and populations behave. That was great because it meant there was already a body of theory that we could lean on to build our models without necessarily starting from a blank sheet and just looking at data.
So the first thing we do to build a model is to try to ask: to what extent the system that you’re interested in is understood, from a theoretical point of view, and then we build a model that reflects that knowledge. And only at that point would we start bringing in data and AI. I would maybe call this ‘theory informed AI,’ which is not the same as what the vast majority of AI companies are doing today. The really interesting bit is this allows us to give insights into potential futures that don’t have a large body of data from part occurrences… which covers many of the most exciting world developments today regarding green/renewable energy, major infrastructure overhauls, smartcities, new government policies, and more.
Q: In the simplest terms, how on earth do you train a model?! In other words, where do you start and what’s the process?
I’ll explain this in terms of how we built Skyral’s Social Modelling Framework. We started by getting familiar with the state of the art in social modelling and experimental psychology. This foundation allowed us to create models and gather data on universal human traits and behaviours that we believed would apply across various scenarios, regardless of cultural, geographical, or social differences. We then built our models and tools around these core features.
These base models serve as a starting point, which can be fine-tuned and improved for specific use-cases by incorporating relevant contextual information about the target group or population.
Think of our framework as a versatile toolkit – a collection of models, data, and tools that you can use to simulate real-world scenarios. However, to create a useful simulation, you need to account for context, including social and cultural factors. For instance, people in London behave differently from those in Sicily or Tokyo. So after we’ve developed a core model that works across different geographical, cultural, and economic contexts, we need to do some more scientific and engineering work to tailor it to the customer’s specific use case. It’s about adapting the model to make it useful for their particular needs.
To make a simulation useful, you need all this contextual information. To make a simulation fast, affordable, and delivered at the speed of relevance – you need generic models, tooling and data – layer on the scenario-specific context… that is what we do at Skyral.
Q: How do you make an AI model ‘smarter?’
Similar to the above, it’s the relevant scenarios applied to the generic model that would make it, colloquially, ‘smarter.’ As an analogy, think of a marble sculpture. An artist starts with a block of marble and roughly carves out a human form. This basic human shape represents our generic, core model. Then they’ll keep chisling away and add specific details – facial features, muscles, clothing – to bring the statue from a generic human shape to take the form of a specific person. These details are the additional data, configurations, and assumptions that we incorporate to tailor the model to a specific use-case or context.
Q: What are the pitfalls one might fall into when training and developing new models?
One major challenge in our case is data. There is not much data available on how people behave in various scenarios. If there was enough data of people’s behaviour in our contexts of interest, we would just use AI, in the sense that most people consider AI, and ask how people behave in a given situation, right? But there isn’t much data that we can use because even if we find data on people’s behaviour, to inform our models we’d also need data on the context in which this data was collected and the stimuli that a person was exposed to. This can be done in laboratory environments and under a lot of constraints, which makes it challenging to inform models of large human populations in the real world.
The follow-on pitfall from not having enough data, is not having reliable data. We have a tendency to over-complicate our problems. As you know, we model humans and we model populations. Sometimes that leads to us acting on the instinct of modelling everything we know about a human. The problem is that we are ourselves humans, which means we are modelling ourselves, right? If you were modelling a strange animal you would only rely on observations. But when you’re modelling yourself, you tend to introspect. You can say, ‘oh, yeah, I definitely need to model this kind of sentiment. I definitely think this kind of behaviour will occur.’ But often, you actually don’t, and in doing so, you’re adding an unnecessary level of complexity. Remember what I said at the beginning? The goal is to find the right level of complexity, to find the model that tells you something useful, but is not so complex that it can’t be validated.
Q: What is the ‘dream state’ of the ‘perfect’ model?
So I would say that the best model is a model that captures the information that tells you something interesting about the system you are looking at – allowing you to make statistically better decisions, while keeping the complexity to minimum.
For example, think of a map. There isn’t a ‘perfect’ map, right? A ‘perfect’ map is the one that gets you where you need to go. If you’re hiking, it might be a topographical map. If you’re in a city, you need a map with the latest roads and landmarks. We want to make ‘maps’ that allow our users to get where they are going the best way possible – without confusion, without unnecessary details – just enough… not too much.
Q: What technologies/engineering breakthroughs are coming to the fore right now that have you really excited?
There is some work being done that I think is very interesting on Automated Scientific Discovery, which is a sub-branch of generative AI. Basically, it’s AI tooling based on Large Language Models (LLM)s that is aimed at supporting, or even conducting, fully automated scientific discovery. So take the body of knowledge a scientist might be interested in, this technology helps them brainstorm new ideas by crunching articles and scientific theories over and over again. I love the idea that machines can help us formulate new questions. And of course, we still need us, because machines can’t conduct experiments (yet!) so I’m really excited to see where that technology will go.